Expectations are that seismic processing projects should be completed faster, so how do we meet the challenge? The answer is complex, however, modifications and manipulations to established systems could enable reductions in turnaround, an example being in building depth imaging velocity models. A model is used to provide an image of the subsurface, from which a range of probabilities and estimates may be made, drilling campaigns planned and then actioned. Seismic data underpins this, and the data’s spatial veracity is dependent on the model used to position the data. Although other factors are important, a seismic processing project is all about the earth model.
Read more in the June edition of First Break 'An innovative approach to automation for velocity model building'.
Automated Models Equivalent to Conventional
Determining design features from one process to apply in another way for a different process is the cornerstone of reverse engineering. A usable velocity model was generated using a Monte Carlo simulation and proves that this type of automation without manual intervention can help reduce turnaround. The result is equivalent to a ‘conventional’ velocity model building exercise.
The starting point for the automation of the velocity model begins by determining how the data supports the model prior to creating a randomly generated model population. Once created, the population is tomographically inverted. A statistical analysis is performed on all the resulting models in the population, and the analysis is used to update the starting point prior to reintroducing a pass of random model generation and inversion. The process is repeated with the goal of producing a model that explains the data, by producing flat Common Image Gathers (CIGs). Move-out metrics are generated after each pass of the simulation, which are used to map the progressive convergence of the solution using these metrics.
An initial model was created to run the test. The model was modified to incorporate a known and locally varying error of up to 10 % in the model. Once randomly perturbed, the starting model was up to 15 % too fast or slow. The results were checked against a final tomographic model which was built using the same data, and generated in 90 days.
The figure below shows three sets of CIGs and three stacks with their associated velocities co-rendered on the seismic sections. The middle image is the result of the 90 day model building exercise. The left hand image shows the starting point for the automated Monte Carlo model building process. The starting CIGs show a significant level of move-out as the model was up to 15 % wrong. The result on the right hand side shows the product of the automated simulation. Gather flatness is equivalent to the conventional approach (middle image), and the co-rendered velocity model closely resembles the model built in 90 days.
A Future of Faster Velocity Model Building
The automated approach to building a velocity model using a Monte Carlo simulation converges on a solution of equal quality to conventional model building. In this example, the starting point was up to 15 % in error, but was still resolved. The workflows were initiated by a geophysicist who had no prior knowledge of the data. No well constraints were available to confirm the accuracy of any of the resulting models. The implications of this approach are considerable. Whilst the original model building took 90 days, the automated model was built in significantly less than an order of magnitude of that time. With the addition of more nuanced automation steps and simplistic machine learning initiatives we anticipate a further reduction the turnaround, the aim being to create an automated model in days rather than months.
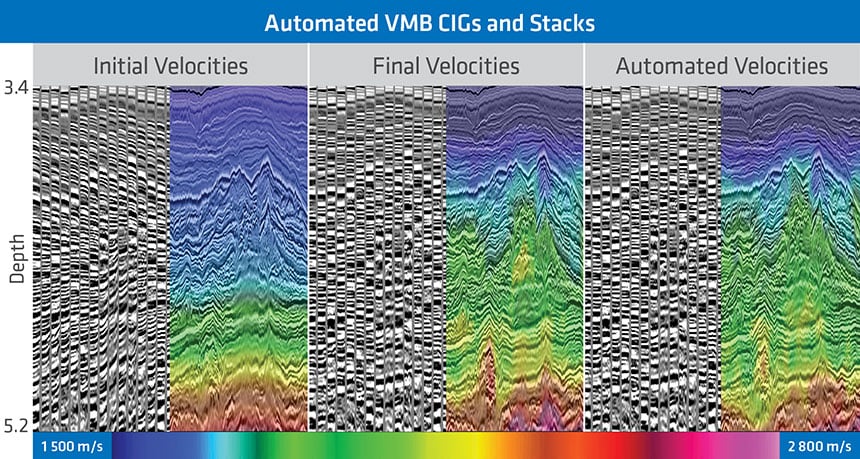
Contact a PGS expert
If you have a question related to our Imaging & Characterization services or would like to request a quotation, please get in touch.